Optimal Post-Hazard Building Reconnaissance
This focus area started from a hypothesis inspired by Dr. Bill Iwan (read a brief summary of his remarkable contribution here). Together with Reza Sheibani, a Ph.D. student who worked on this topic, we hypothesized that, due to the similar material used in construction, similar geometries of building structures, and similar building codes governing the designs and retrofits of building structures, certain patterns in damage intensities of buildings will emerge during a seismic event. Utilizing only a small sample set that incorporates the ground motion information and necessary structural attributes, such patterns can be learned and the damaged condition of every structure in the affected region can be inferred.
Following the hypothesis, the data-based damage inference framework is proposed first for a seismic event and validated on two regional simulation testbeds (later became the R2Dtool) developed by NHERI SIMCENTER. With a selection of only 500 buildings, we were able to infer the damage conditions of 20,000 buildings in a seismic event scenario, wherein more than 90% of the buildings had an estimated maximum drift error of less than 10%, compared to the testbed results.
Read more about our work here.
RELATED RESEARCH PROJECT:
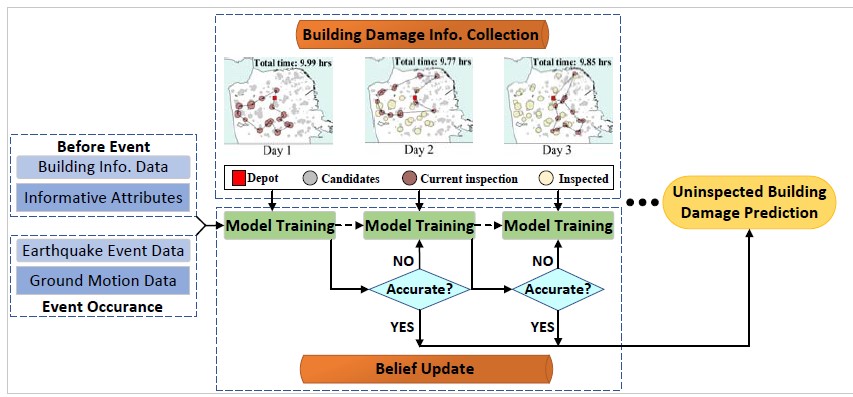
This project supports fundamental research to develop a mathematical modeling framework to guide inspection teams through post-seismic reconnaissance missions. This new approach will holistically identify buildings to be prioritized for inspection and design inspection schedules to efficiently visit these buildings with limited time and inspection crew members. This research integrates concepts from statistical and optimal learning with models for routing and scheduling. These two aspects have been extensively studied separately, but never jointly. This knowledge gap poses a serious challenge to the guidance of inspection teams, which collect information in the field subject to resource constraints. The research team will develop an integrated modeling framework that bridges optimal learning and combinatorial optimization to identify inspection routes and schedules that maximize the predictive power of machine learning models for post-seismic building damage assessment.